How AI is transforming predictive analytics in finance
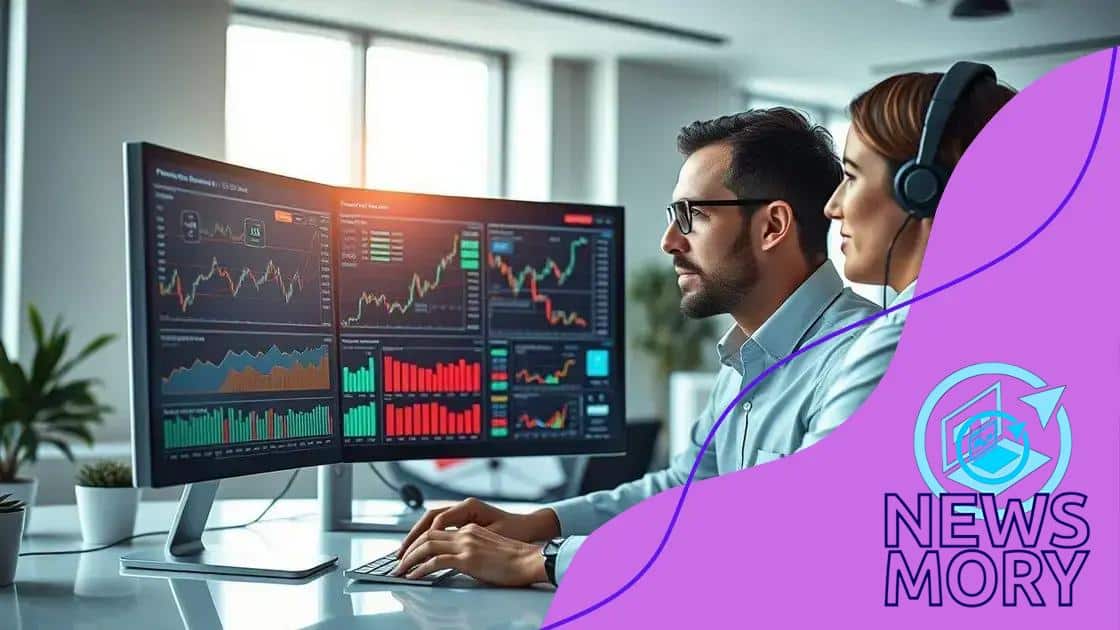
AI is transforming predictive analytics in finance by enhancing risk assessment, personalizing customer experiences, and enabling real-time data analysis, thereby allowing institutions to make informed decisions and improve operational efficiency.
How AI is transforming predictive analytics in finance is a pressing question for industry leaders today. As financial institutions seek to enhance decision-making, understanding AI’s role becomes crucial. Have you considered the potential insights waiting to be uncovered?
Introduction to predictive analytics in finance
Understanding predictive analytics in finance is essential for organizations aiming to make informed decisions. It involves using data to forecast outcomes and trends that can directly impact financial success. With advanced technologies, businesses can adapt quickly to market changes.
The Basics of Predictive Analytics
At its core, predictive analytics relies on statistical algorithms and machine learning techniques to analyze current and historical data. This process helps identify patterns that can predict future events. Financial institutions leverage these insights to enhance their strategies.
- Improves forecasting accuracy
- Enhances risk management
- Identifies customer behaviors
- Optimizes operational efficiency
By utilizing predictive models, companies can better understand potential risks and opportunities. For example, they can analyze customer spending habits to tailor marketing approaches effectively. Moreover, this data-driven method reduces uncertainty and allows for proactive measures.
Why Financial Institutions Are Investing
Many financial institutions see predictive analytics as a necessary investment for future growth. This technology enables them to stay competitive by making data-driven decisions that lead to improved profitability. As a result, the demand for skilled analysts is increasing within the industry.
Alongside analytics, evolving technologies like artificial intelligence (AI) are enhancing predictive capabilities. AI allows for more complex data processing, resulting in deeper insights into consumer behavior and market fluctuations.
- Greater scalability of solutions
- Enhanced customer experience
- Faster, data-driven decision-making
- Reduction of operational costs
As finance continues to evolve with technology, understanding predictive analytics will prove essential for firms looking to succeed in a dynamic environment. Embracing these innovations will empower institutions to make informed choices that align with current market trends.
Key AI technologies driving change
Several key AI technologies are transforming the landscape of predictive analytics in finance. These advancements enable financial institutions to leverage vast amounts of data for better insights and decision-making.
Machine Learning
Machine learning is at the forefront of AI transformations. It allows systems to learn from data without explicit programming. Algorithms improve their performance as they are fed more data over time.
- Identifies patterns and anomalies
- Predicts future outcomes based on historical data
- Enhances risk assessment capabilities
Machine learning is essential for detecting fraudulent transactions and foreseeing market shifts. As models learn, predictions become more accurate, allowing institutions to react swiftly.
Natural Language Processing (NLP)
Natural Language Processing plays a significant role in understanding and interpreting vast amounts of unstructured data. It can analyze customer feedback, market reports, and news articles in real-time.
- Improves customer interaction through chatbots
- Analyzes sentiment to assess market conditions
- Extracts insights from financial documents
The ability of NLP to process language efficiently means financial firms can gain deeper insights into market trends and customer needs. This analysis improves strategic planning and customer engagements.
Robotic Process Automation (RPA)
Robotic process automation enhances operational efficiency by automating repetitive tasks. By handling data entry and basic analysis, RPA frees up valuable time for analysts to focus on strategic decision-making.
- Reduces human error in data processing
- Streamlines operational workflows
- Increases compliance and regulatory adherence
The efficiency brought by RPA is particularly important in finance, where accuracy and speed are critical. It allows for quicker reporting and analysis, helping firms remain competitive.
In summary, these key AI technologies are not only driving predictive analytics forward but also shaping the future of the financial industry. By integrating these tools, firms can harness data more effectively and respond proactively to ever-changing market dynamics.
Impact of AI on risk assessment
The impact of AI on risk assessment is profound in the financial sector. By integrating advanced technologies, organizations can evaluate risks more accurately and efficiently. This capability allows them to identify potential issues before they escalate into serious problems.
Enhanced Predictive Capabilities
AI uses data analytics to improve predictive capabilities. By analyzing historical data and current market trends, AI models can predict potential risks more effectively. This not only helps in managing financial risks but also in understanding customer behaviors.
- Identifies potential credit risks
- Monitors real-time market fluctuations
- Assesses risk exposure across various portfolios
Financial institutions can leverage these insights to develop strategies that minimize risks. By employing machine learning algorithms, banks can continuously improve their risk assessment models.
Fraud Detection
AI significantly enhances fraud detection systems. Traditional methods often rely on predefined rules, which can miss new threats. However, AI algorithms can adapt and learn from new data.
- Recognizes unusual patterns in transactions
- Improves response time to potential fraud
- Reduces false positives in fraud alerts
This adaptability allows organizations to stay one step ahead of fraudsters. It gives them the ability to protect their assets and maintain customer trust in their services.
Furthermore, AI’s continuous monitoring capabilities ensure that risks are assessed in real-time. This level of vigilance is essential for mitigating losses caused by unexpected events. The integration of AI technologies into risk assessment processes empowers financial institutions to build stronger defenses against various risks.
Real-world examples of AI in finance
Exploring real-world examples of AI in finance showcases how innovative technologies enhance operational efficiency and decision-making. Various financial institutions have successfully implemented AI solutions to improve services and customer experiences.
JP Morgan Chase
JP Morgan Chase uses AI technology in its contract review process. Their AI platform, called COiN (Contract Intelligence), analyzes legal documents and extracts important data. This system can review thousands of contracts in seconds, saving time and reducing human error.
- Enhances contract management efficiency
- Reduces operational costs
- Minimizes risks associated with manual reviews
By automating these tasks, JP Morgan Chase allows their employees to focus on more complex issues, leading to better resource allocation.
Goldman Sachs
Goldman Sachs leverages AI in wealth management through its Marcus platform. AI algorithms analyze customer data to create personalized financial advice. By assessing market trends and individual preferences, Marcus provides clients with tailored investment options.
- Improves customer satisfaction
- Offers personalized financial services
- Enhances investment strategies
This personalized approach enhances customer engagement and builds long-term relationships with clients.
Bank of America
Bank of America utilizes AI with its virtual assistant, Erica. This chatbot helps customers with account inquiries, payment reminders, and financial advice. By using natural language processing, Erica can understand and respond to customer queries in real-time.
- Provides 24/7 customer support
- Reduces response time for inquiries
- Enhances customer interaction through technology
By integrating Erica, Bank of America improves user experience and operational efficiency, showcasing how AI can transform customer service in finance.
These examples highlight how major financial institutions harness AI to innovate and improve their offerings. As AI technology evolves, its role in finance will expand, leading to even greater advancements.
Future trends in financial analytics
As the landscape of finance evolves, several future trends in financial analytics are emerging. These trends indicate how organizations will leverage data and technology to stay competitive in an ever-changing environment.
Increased Use of Artificial Intelligence
Artificial intelligence is set to play a pivotal role in financial analytics. Companies are investing in AI to automate data analysis and gain deeper insights. This technology will help them make faster and more informed decisions.
- Streamlines data processing
- Enhances predictive accuracy
- Improves risk assessment capabilities
As AI technologies continue to advance, financial institutions will adopt smarter algorithms to predict market changes. This will enable them to react promptly and strategically to shifts in the economy.
Integration of Big Data
The integration of big data into financial analytics is another significant trend. With vast amounts of data being generated daily, organizations must take advantage of this information to drive their strategies.
- Identifies customer trends and behaviors
- Optimizes portfolio management
- Enhances compliance and regulation tracking
By analyzing big data, companies can identify new opportunities and better understand their clients’ needs. This aspect will be crucial for developing tailored financial products and services.
Rise of Real-Time Analytics
Real-time analytics are becoming increasingly important in finance. Organizations need instant access to data to make quick decisions. Utilizing technology to monitor financial performance continuously is essential for success.
With real-time analytics, financial institutions can respond to market fluctuations as they happen, allowing them to capitalize on opportunities or mitigate risks immediately. This agility is vital in today’s fast-paced financial landscape.
As these trends continue to evolve, the financial sector will see a greater reliance on technology and data-driven insights. Embracing these changes is essential for companies looking to thrive in a competitive market.
FAQ – Frequently Asked Questions about AI in Finance
How is AI improving financial decision-making?
AI enhances financial decision-making by analyzing data quickly and accurately, allowing institutions to identify trends and make informed choices.
What role does big data play in finance?
Big data helps financial institutions uncover valuable insights about customer behavior and market trends, leading to better services and strategies.
How does AI contribute to risk assessment in finance?
AI improves risk assessment by providing real-time analysis and predictive insights, allowing companies to identify potential risks before they become critical.
What is the future outlook for AI in financial analytics?
The future of AI in financial analytics looks bright, with increasing reliance on real-time data and automation to drive decision-making and operational efficiency.