The role of machine learning in modernizing healthcare systems
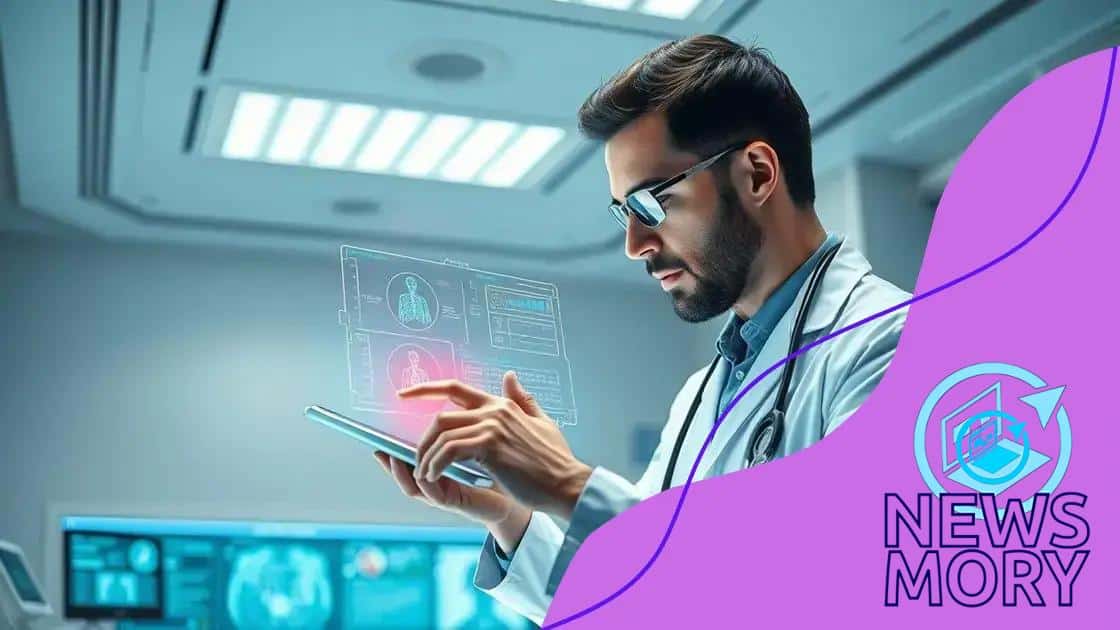
The role of machine learning in modernizing healthcare systems enhances patient care through predictive analytics, personalized treatments, and improved operational efficiency, while addressing challenges like data privacy and integration with existing infrastructure.
The role of machine learning in modernizing healthcare systems is becoming increasingly significant. Have you noticed how technology has shaped your doctor visits or hospital stays? Let’s dive into this fascinating topic and uncover its impact.
Understanding machine learning’s basics in healthcare
Understanding machine learning basics in healthcare is crucial for grasping how technology enhances medical practices. It opens the door to innovative solutions, making patient care more effective.
What is Machine Learning?
At its core, machine learning is a subset of artificial intelligence that allows computer systems to learn and make decisions based on data. Unlike traditional programming, where explicit instructions are given, machine learning enables systems to improve through experience.
Key Components of Machine Learning in Healthcare
- Data collection: Accumulating vast amounts of patient data from diverse sources.
- Algorithms: Using complex mathematical models to analyze and predict health outcomes.
- Training: Continuously feeding data to the system so it learns over time.
This process fosters better decision-making and insights into patient care. With each interaction, machine learning systems adapt and become more proficient at identifying patterns and anomalies.
Healthcare professionals now utilize machine learning for various applications. For instance, it aids in screening diseases and personalizing treatment plans. By analyzing patient histories, algorithms can highlight risks that might be overlooked.
Real-World Impact
The impact of machine learning on healthcare is evident in numerous success stories. For example, hospitals are employing these technologies to reduce wait times and improve diagnosis accuracy. As systems refine their predictive capabilities, outcomes for patients continue to improve.
These advantages don’t come without challenges. Ensuring data privacy and security remains a top priority as healthcare providers implement machine learning. Nevertheless, addressing these concerns can pave the way for more widespread adoption.
Key benefits of machine learning for patient care
The key benefits of machine learning for patient care are transforming how healthcare professionals enhance their practice. By leveraging advanced technology, hospitals and clinics can deliver more accurate and personalized services.
Improved Diagnostics
One significant advantage of machine learning is its ability to improve diagnostics. Algorithms can analyze medical images, lab results, and other data with higher accuracy than traditional methods. This capability leads to earlier detection of diseases, allowing for timely treatment.
Personalized Treatment Plans
Additionally, machine learning enables the creation of personalized treatment plans. By examining patient history and outcomes, algorithms can suggest the most effective therapies tailored to individual needs. This personalized approach often results in better patient outcomes.
- Enhanced accuracy in disease prediction.
- Customized therapy options based on unique patient profiles.
- Improved adherence to treatment regimens through timely interventions.
The integration of machine learning also helps in managing chronic conditions. Patients with ongoing health issues benefit from continuous monitoring and predictive analytics. For instance, systems alert healthcare providers about potential complications before they become critical.
Operational Efficiency
Another essential benefit is operational efficiency. Machine learning can streamline administrative tasks, such as appointment scheduling and billing. This efficiency allows healthcare providers to focus more on patient care rather than paperwork.
Moreover, by analyzing vast amounts of data, machine learning systems identify patterns that can enhance resource allocation. This means hospitals can optimize their use of staff and equipment, ensuring that care is both effective and efficient.
Enhanced Patient Engagement
Lastly, machine learning fosters enhanced patient engagement. Tools that leverage AI help patients manage their health proactively. For example, chatbots can answer common queries and provide information about treatment plans.
Challenges in implementing machine learning in healthcare
While the potential of machine learning in healthcare is vast, several challenges hinder its implementation. Addressing these obstacles is crucial for realizing the benefits of this technology.
Data Privacy Concerns
One significant challenge is data privacy. Healthcare organizations collect sensitive patient information that must be protected. Ensuring compliance with regulations like HIPAA is essential, but can complicate data sharing and analysis.
Integration with Existing Systems
Another issue is the integration of machine learning systems with existing healthcare infrastructure. Many hospitals use legacy systems that may not easily support new technologies. This incompatibility can lead to data silos, where valuable information is isolated and not leveraged effectively.
- Compatibility with electronic health records (EHR).
- High costs associated with upgrading systems.
- Need for training personnel on new software.
Moreover, there is a need for significant investment in technology and talent. Hiring skilled data scientists and engineers can strain budgets, especially for smaller institutions. Upfront costs may discourage some organizations from adopting machine learning.
Quality of Data
The quality of data is another major challenge. Machine learning algorithms depend on accurate and comprehensive datasets. Poor or biased data can lead to unreliable predictions and outcomes, potentially compromising patient safety.
For instance, if the data used for training algorithms does not represent diverse patient populations, the results may not be applicable to everyone. This lack of inclusivity could widen health disparities instead of narrowing them.
Change Management
Adapting to change is vital for successful implementation. Healthcare professionals must be open to revising their workflows and embracing new technologies. Resistance to change can stall progress and delay the benefits that machine learning can provide.
Real-world examples of machine learning success
Real-world examples of machine learning success in healthcare illustrate its powerful impact on patient outcomes and operational efficiency. These stories showcase how organizations are harnessing this technology to enhance care.
Predictive Analytics in Patient Care
One notable example is the use of predictive analytics to manage patient care more effectively. Hospitals apply machine learning algorithms to analyze historical health data. This analysis helps identify patients at risk for conditions such as heart attacks or readmissions.
- By flagging high-risk patients, healthcare providers can intervene earlier.
- Implementing tailored treatment plans reduces hospital stays and costs.
- Patient outcomes improve significantly through timely, proactive care.
This approach has yielded impressive results in various healthcare settings, demonstrating that predictive analytics can save lives.
Machine Learning in Radiology
Another successful application of machine learning is in the field of radiology. Advanced algorithms assist radiologists in interpreting medical images, such as X-rays and MRIs. These systems help detect abnormalities that might be missed by the human eye.
For example, AI tools can identify early signs of diseases like cancer, allowing for earlier intervention. As a result, patient survival rates have significantly improved. These systems not only boost diagnostic accuracy but also enhance workflow efficiency in radiology departments.
Improving Operational Efficiency
Organizations are also using machine learning to improve operational efficiency. For instance, hospitals utilize AI to optimize scheduling, ensuring that staff and resources are allocated effectively. Predictive scheduling maps patient volumes, allowing for better planning.
This optimization reduces wait times, increases patient satisfaction, and maximizes the use of facilities. Additionally, machine learning aids in inventory management by predicting supply needs, helping to avoid shortages.
Telemedicine and Remote Monitoring
Telemedicine has also benefited from machine learning advancements. AI-driven platforms analyze patient data collected through wearable devices, offering real-time insights. This capability supports continuous patient monitoring and timely interventions.
Such innovative solutions have allowed healthcare providers to maintain connections with patients, even remotely, improving adherence to treatment plans and enhancing overall health outcomes.
Future trends of machine learning in healthcare
The future trends of machine learning in healthcare promise to reshape the way we approach medical treatment and patient care. As technology continues to advance, several key developments are on the horizon.
Increased Personalization
One major trend is the shift towards increased personalization in healthcare. Machine learning algorithms will refine their ability to analyze patient data, leading to more tailored treatment plans. This means that patients will receive therapies specifically suited to their genetic makeup and lifestyle.
- Advanced genomics will guide personalized medicine.
- Machine learning will assess individual responses to treatments.
- Proactive health management strategies will emerge based on personal data.
This level of customization aims to improve patient outcomes and ensure that each person is treated as a unique individual.
Integration with IoT Devices
The integration of machine learning with Internet of Things (IoT) devices will also be a game changer. As more patients use wearable devices to monitor their health, machine learning will process this data in real-time.
These insights will enable healthcare providers to detect issues early and respond promptly. For example, wearable sensors can alert medical professionals about irregular heart rates or potential falls in elderly patients, allowing for immediate intervention.
Advancements in Telehealth
Another trend to watch is the advancements in telehealth solutions. With the rise of remote consultations, machine learning will enhance telemedicine platforms by improving diagnostic accuracy and patient engagement.
Virtual assistants, powered by AI, will be able to manage patient inquiries, schedule appointments, and provide health information seamlessly. This will not only improve efficiency but also create a better overall patient experience.
Ethical AI and Bias Mitigation
As machine learning becomes more prevalent, addressing ethical concerns will be essential. Future trends include developing ethical AI frameworks to ensure fairness and reduce biases in healthcare applications.
By focusing on diverse datasets and transparency in algorithm development, the industry can minimize health disparities. The goal is to create machine learning models that serve all populations equitably, enhancing trust in AI solutions.
FAQ – Frequently Asked Questions about Machine Learning in Healthcare
How does machine learning improve patient care?
Machine learning analyzes vast amounts of patient data to provide insights, leading to earlier diagnoses, personalized treatments, and better health outcomes.
What are the privacy concerns with machine learning in healthcare?
Data privacy is critical, as sensitive patient information must be protected to comply with regulations like HIPAA while still allowing effective data analysis.
How can machine learning help with predictive analytics?
Machine learning algorithms can identify patients at risk of developing conditions, enabling healthcare providers to intervene earlier and improve patient safety.
What future trends should we expect in healthcare with machine learning?
Future trends include increased personalization of treatments, better integration with IoT devices, advancements in telehealth, and addressing ethical concerns in AI.